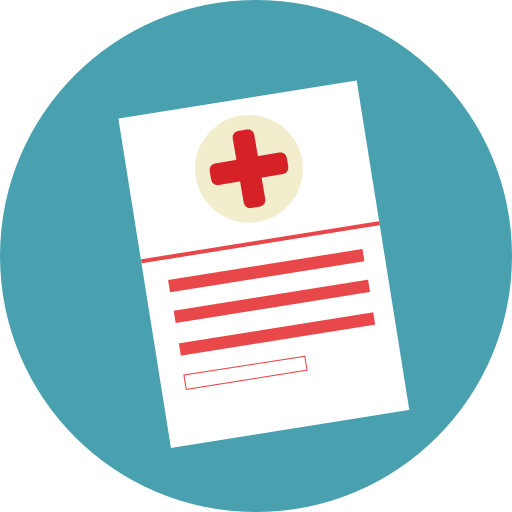
International Journal of Clinical and Medical Case Reports
Department of Clinical Laboratory, Beijing Chest Hospital, Capital Medical University, Beijing Tuberculosis and Thoracic Tumor Research Institute, No.9 Beiguan Street, Tongzhou District, Beijing (101149), China 2Department of Medical Oncology, Beijing Chest Hospital, Capital Medical University, Beijing Tuberculosis and Thoracic Tumor Research Institute, Beijing(101149), China. 3Laboratory for Clinical Medicine, Capital Medical University, No.9 Beiguan Street, Tongzhou District, Beijing, China
Immune checkpoint inhibitors (ICIs) hold a great promise in treatment of non-small cell lung cancer (NSCLC), while only a portion of patients benefited from the treatment, and others could not achieve optimal therapeutic effects from initial immunotherapy, even for those patients with PD-L1 tested positive. However, the clinical markers for the selection of patients who will benefit from ICIs treatment before hand are largely unknown. In this study, a retrospective analysis was conducted on baseline information of 144 patients with advanced/metastatic NSCLC who received ICIs treatment from the November of 2018 to the January of 2023
GLB •ANC •Bone metastasis •ICIs • Advanced NSCLC • Prognosis
Lung cancer takes the leading position in human cancers in terms of incidence and mortality worldwide [1, 2]. Non-small cell lung cancer (NSCLC) accounts for 80~85% of all lung cancer cases in the Word [3]. Theapplication of immune checkpoint inhibitors (ICIs) to immunotherapy in lung cancerled to striking improvement in progression-free survival (PFS) and overall survival (OS) of NSCLC patients[4-6] . Despite the increasing role of immunotherapy in lung cancer, a minority of patientsstill could not benefit from these therapies. Furthermore, the occurrence of immunotherapy-related toxic effects in some patients diminishes the quality of life [7-10]. Thus, it becomes imperative todetermine clinical biomarkers, by which the patients who will benefit from ICIs could be hopefully differentiated from those who will not, to avoid unnecessary treatment. PD-L1 was the first FDA-approved predictive biomarker for (NSCLC) in 2015, and series of studies have also obtained this outcome [11, 12]. Moreover, tumor mutational burden (TMB) and microsatellite instability-high (MSI-H) can also serve as effective predictive indicators of prognosis in these patients, andother indicators such as circulating tumor DNA (ctDNA) and circulating tumor cells (CTC) have shown promise in prognostic prediction [13, 14] . However, there are some limitations and disadvantage of using these markers, for example, examination of these markers is expensive, and inconvenient to perform. An easily predictive model is urgently required in clinical practice to accurately guide clinical treatment for NSCLC. Currently, the predictive model of ICIs for NSCLC treatment has much room for improvement. Some investigators suggested the utilization of the Lung Immune Prognostic Index (LIPI), a composite of lactate dehydrogenase (LDH) and neutrophil-associated ratio (dNLR), to predict prognostic outcome[15, 16]. T cell-associated immunoglobulins may be related to the phenomenon of antibody neutralization, where immunoglobulins may bind to immune checkpoint inhibitors (ICIs) or other immunotherapeutic drugs, thus inhibiting their activity and reducing treatment effectiveness [17]. However, the predictive model of ICIs combined therapy was not so effective as expected, and the clinical value of the predictive model that integrates the clinical characteristics and laboratory indicators of patients before treatment deserves further validation. To improve the prognostic survival of patients treated with ICIs and select suitable groups of patients by predictive biomarkers for ICIs treatment, we conducted this study to develop a non-invasive and cost-effective multivariable prognostic model. We speculated that a model that integrated clinicopathological characteristics and laboratory indicators could simultaneously predict the survivals of NSCLC patients that received ICIs combined therapy.
Total 144 patients with advanced NSCLC (III/IV) who received immune checkpoint inhibitor therapy between November 2018 and January 2023 were retrospectively analyzed. Data were collected from the records of hospitalized. This study was approvedby the Beijing Chest Hospital Medical Ethics Committee (YJS-2023-09), according to the local regulations and the Helsinki declaration. A waiver for patients’notice consent was obtained by the Beijing Chest Hospital Medical Ethics Committee due to the retrospective nature of the study.
Adult patients aged 18-80 years and diagnosed as advanced NSCLC (IIIB/ IIIC/IV) by pathology were included in this study if theydid not receive surgical operation and radical synchronous or sequential radiotherapy and were treated with immune checkpoint inhibitors at least two cycles with complete medical records and follow-up records . Of note, those patients who were diagnosed with other tumors and infectious diseases, treated with steroid hormone for 1 month before treatment, or diagnosed with hematological or autoimmune diseases, were excluded from this study.
All ICIs were approved by National Medical Products Administration (NMPA) in China. The dosage and frequency of immune checkpoint inhibitors used in clinical trials are recommended according to the manufacturer’s instructions.
The time of the first ICIs treatment was recorded as the starting point of observation, and each patient was followed up with interviews by telephone, outpatient review, and other forms of visit. Follow-ups were performed until disease progression or decease, and the follow-up study ended on December 25, 2023. Overall survival is the time from the start of immunotherapy to decease or the last follow-up visit. Progression-free survival (PFS) was defined as the time from the start of immunotherapy to clinical or imaging progression
The clinical indicators collected by electronic medical record system included: Age, gender, smoking history, clinical stage, ECOG PS score, histology, therapeutic regimen, PD-L1 expression, and metastasis for tumor progression. The baselines were defined as the measurements taken within 1 week prior to the treatment with ICIs. The baseline data of peripheral blood included WBC (white blood cell count), ANC(Absolute neutrophil count), LY (Lymphocyte count), NLR (Neutrophil to lymphocyte ratio), PLR (Platelet to lymphocyte ratio), CRP (C-Reactive Protein), SII (Systemic Immune Inflammation Index), MLR (Monocyte to lymphocyte ratio), LDH (lactic dehydrogenase)), GLB (serum globulin), A/G (serum albumin to globulin ratio). A flow chart of sample screening and model constructionwas shown in Figure 1.
Data processing was conducted using SPSS 27.0 software. For descriptive analysis, the normal and non-normal distributions of continuous variables were expressed by mean ± standard deviation (SD) and median [interquartile range (IQR)], respectively, while categorical variables were presented as percentages. X-tile softwarewas utilized to determine the optimal cut-off value and divides continuous data into categorical variables. Lasso regression was used to screen for the risk factors. Prediction models including parameters screened by Lasso regression were established based on Cox regression. The predictive abilities of models were compared by C-index. Graphpad prism10.0 for Kaplan Meier method was used to calculate PFS and OS curves, and log-rank test to evaluate differences. The survival, rms, glmnet packages, etc. from Rstudio (version 4.3.2) were used to draw clinical decision curves, column charts for prediction of survivals, and Lasso regression plots. A P value < 0.05 was considered statistically significant. Achart of model construction was shown in Figure 2
This study included a total of 144 patients (109 males, and 35 females) with advanced/metastastic NSCLC (Table 1). Among these patients, 47 were ≤ 60 years old, and 97 > 60 years old. According to clinical stages, 38 patients in stage III and 106 in stage IV. There were 82 cases of adenocarcinoma and 62 cases of squamous cell carcinoma. 100 patients had a smoking history. All patients were treated with PD-1 or PD-L1 immune checkpoint inhibitors. Most people have an ECOG score of 0-1 (137/144, 95.1%). Patients with the distant metastases suffered from bone metastases in most cases (38/144,26.3%). Overall, the median PFS and median OS were10.7 and 30.0 months, respectively.Survival curves of immunotherapy for advanced lung cancer were shown in Figure 3a&b.
There were 82 cases of adenocarcinoma and 62 cases of squamous cell carcinoma.As shown in Figure 4a, the median PFS of the two groups of patients were 10.8 and 10.7 months respectively, with the HR=0.878 (95%C1: 0.667-1.156) and P=0.354, suggesting that the difference between the two groups of patents were not significant. As shown in Figure 4b, the median OS were 33.7 and 25.3 months respectively, with the HR=1.146(95%CI:0.906-1.449) and P=0.257, and there was no difference between the two groups of patients. These data indicate the survivals of patients with adenocarcinoma and squamous cell carcinoma is similar. Interestingly, when analyzing102 cases with negative PD-1 expressionand 42 cases with positive PD-1 expression via PFS and OS,we found that the median PFS of the two groups of patients were 9.0 and 14.2 months respectively, with HR=1.732(95%C1:1.108-2.708) and P=0.016 (Figure 4c), suggesting that the PFS of patients with PD1 expression was greater than that of patients without PD1 expression. Consistently, the median OS of the two groups of patients were 23.4 and 38.5 months respectively, with HR=2.432 (95%C1:1.483-3.988) and P< 0.001 (Figure 4d), indicating that patients with PD1 expression survived much longer than the ones without PD1 expression. These data suggest that PD1 expression in patients affects the efficacy of ICIs treatment. (a&b) PFS and OS curves of patients with Adenocarcinoma and Squamous cancers as examined by histology. (c&d) PFS and OS curves of patients without (negative) or with (positive) PD-L1 expression. X-tile software was used to calculate the optimal cut-off value for PFS and OS, dividing peripheral blood markers into high and low groups. Their cut-off values were: NLR>3, LMR>2.4, SII>979, GLB (g/L)>26.6, A/ G>1.4, WBC (10 9/L)>6.4, LY (10 9/L)>1.5, and ANC (10 9/L)>5. No optimal cut-off values were determined for PLR,CRP (mg/L), and LDH (U/L).These cut-off values can be used to predict the survivals of the NSCLC patients with ICIs treatment. Determination of OS and PFS by univariant, lasso, and multivariate analysis of biomarkers. The results of univariate Cox regression analysis show that a total of 10 univariate factors, including clinical stage, ECOG score, bone metastasis, LMR, SII, GLB, A/G, WBC, LY, and ANC, had statistical significance in PFS (P< 0.05) , and that a total of 10 single factors, including Histology, ECOG score, treatment methods, bone metastasis, PD-L1 expression in tissues, NLR, SII, GLB, A/G, WBC, and ANC, were statistically significant in OS (P< 0.05). Lasso regression was also used to screen parameters related to OS and PFS. As shown in Figure5a, ten variables related to PFS were included in Lasso regression, and when the model fitted well, seven to 10 independent variables were contained in between (Figure5b). For OS, twelve variables were included in Lasso regression (Figure5c), and when the model fitted well, Two to 10 independent variables were contained in between (Figure5d). After Lasso regression ,these variables would subject to, Cox multivariate analysis.
To select significant variables and Identify factors of statistical significance to both PFS and OS, weperformed Cox multivariate regression, and found that GLB (g/L)>26.6, ANC>5(109/L), and bone metastasis were statistically significant in the multiple regression of PFS and OS (P<0.05,
For the overall population, the medians of OS and PFS demonstrate distinct outcomes based on specific biomarkers. According to the univariate analysis, the factors of statistical significance were as follows: GLB: Individuals with a low GLB (≤26.6) had a markedly longer median of PFS (18.8 months) and OS (38.5 months) compared to those with high GLB (>26.6), which had a median of 7.9 months for PFS and median of 22.0 months for OS (Fig. 6a&b). The hazard ratios for PFS and OS were 1.886 (95% CI: 1.250-2.848, P=0.003) and 1.955 (95% CI: 1.279-2.990, P=0.0005) respectively, indicating that patients with low GLB levels survived much better than the ones with high GLB. These data demonstrate that GLB level of patients could serve as a potential biomarker of prognosis forpatients.
Patients with low ANC (≤5) had a longer median of PFS (13.9 months) and OS (38.0 months), whereas those with high ANC (>5) had a median of 7.3 months for PFS and median of 19.4 months for OS (Fig. 6c&d). The hazard ratios were 2.391 (95% CI: 1.528-3.741, P=0.0059) for PFS and 2.101 (95% CI: 1.307-3.379, P=0.0009) for OS. Similar to GLB, ANC level ofpatients might be used as a biomarker of prognosis for patients
The presence of bone metastasis significantly affected survival outcomes, with patients having non-bone metastasis showing a median of 12.1 for PFS months and median of 31.9 months for OS, compared to those with bone metastasis, who showed a median of 7.9 months for PFS and median of 19.1 months for OS (Fig. 6e&f). The hazard ratios were 2.548 (95% CI: 1.557-4.169, P=0.0006) for PFS and 2.098 (95% CI: 1.260-3.492, P=0.0047) for OS (Fig. 6g). Thus, bone metastasis ofpatients can be used as a potential biomarker of prognosis for patients.
Positive PD-L1 expression of patients was associated with a better median of OS (39.8 months) compared to that of patients with negative PD-L1 expression (23.4 months), with a hazard ratio of 1.925 (95% CI: 1.245-2.979, P=0.0009), indicating that PDL1 expression affected OS of the patients. According to the univariate analysis, the levels of GLB, ANC, bone metastasis and PD-L1 expression are highly correlated to the survival of patients, and could be used as a useful biomarkers of prognosis for patients
Based on the number of advantaged factors (i.e., GLB≤26.6 g/L, ANC≤5 * 109/L, no bone metastasis, and PD-L1 expression positive), We explored the OS and PFS (Fig.7a&b). It was found that there was statistical differences among the groups, and that the OS and PFS of Groups A and B significantly increased compared to that of the other groups (C, D, E) (P< 0.0001), suggesting that the survivals of patients increased depending on the points of the prognostic scoring system.
To further evaluate the predictive model and the contribution of each variable to survival, we established a 1-year PFS rate and 1-year OS rate nomogram based on multivariate Cox regression (Figure 8a&b). The C index of 1-year PFS rate was 0.731 (0.648-0.814) (P< 0.001), and the C index of 1-year OS rate was 0.733 (0.635-0.832) (P< 0.001). The results of Decision Curve Analysis (DCA) demonstrate that the nomogram model performed well in clinical practice (Figure 8 c&d).
After internal cross-validation calibration, tested with 5-fold crossvalidation, and repeating the procedure 10 times, the C statistic (AUC under the ROC curve of one-year PFS rate) was 0.808 (95% CI: 0.655- 0.961), and the C statistic (AUC under the ROC curve area of one-year OS rate) was 0.783 (0.633-0.933) (Figure 9 a&b). These datasuggest that the models were reliable
Although it was reported that noninvasive peripheral blood markers and clinical factors had predictive values in the treatment of advanced NSCLC, such as NLR, SII, there is no universal optimal cut-off value determined in different studies in the treatment of NSCLC patients with ICIs, and application of combined clinical markers is rarely seen.[18, 19]. In this study, 144 patients with advanced/metastatic NSCLC were treated with ICIs and analyzed by combined clinical features, organ metastasis, peripheral blood and biochemical indicators. A prognostic score including GLB, ANC, bone metastasis, and PD-L1 expression was determined and validated. The final cumulative score by grouping the four factors can predict survival prognosis of patients. In both OS and PFS, the higher the score, the worse the prognosis, and the difference between groupswith scores by this subgrouping was highly significant (P< 0.001). Compared with the commonly-used continuous peripheral blood variables without generally-recognized optimal cut-off value in most studies, this scoring system would be a much more valuable alternative to clinical guidance. In order to further understand the contribution of each factor to the survival of patients and the clinical application value, we constructed one-year PFS and OS rate nomogram for individual survival prognosis prediction. The DCA curves of one-year PFS and OS rate are effective in clinical practice. The whole area of DCA curve of one-year OS rate indicate that this model is of high clinical relevance (Figure 8d). In terms of model validation, due to the limited sample size, we adopted the internal validation, k-fold cross validation (k=5), which divided the sample size into five subsets for mutual validation of the training set and the validation set. The C statistic of 1-year PFS rate was 0.808, and The C statistic of 1-year OS rate was 0.783, supporting the prediction of the model. These data indicate that GLB, ANC, bone metastasis and PD-L1 expression in patients with advanced/metastatic NSCLC are predictive factors closely-related to ICIs treatment.
Neutrophils play a crucial role in the tumor microenvironment. It was reported that neutrophil account and percentage may correlate with the prognosis of ICIs treatment[20, 21] , and that organ metastasis can predict the prognosis of NSCLC patients treated with ICIs [22, 23] . Therefore, organ metastasis was included as a factor in modeling in this study. Up to now, there are no sufficient data regarding the application of the GLB and ANC in the field of cancer immunotherapy. Our data suggest that ANC, GLB and bone metastasis serve as useful biomarkers that can be employed to select the advanced NSCLC population for immunotherapy. It was reported that serum globulin had prognostic relevance in patients with cancer[24, 25], for example, a retrospective analysis of 186 patients with gastric cancer indicated that a baseline GLB of less than 33.4 (g/L) in patients was a favorable prognostic factor [24] . In addition, a nomogram prediction model established using the baseline peripheral blood data of 336 patients with oral cancer set the optimal cut-off values of GLB as 26.35g/L, which could predict the prognosis of patients [25], and the multivariate result OR=1.093 (1.014-1.177), P=0.019 was close to the GLB cut-off value in the present study (GLB > 26.6). According to our results, abnormal serum is a poor prognostic factor in patients with tumors. Furthermore, this study focused on the patients with ICIs treatment, and we hypothesized that several mechanisms might be involved. First, Antibody neutralization works: the high level of immunoglobulin may be related to the phenomenon of antibody neutralization, i.e., immunoglobulin may bind to immune checkpoint inhibitors or other immunotherapeutic drugs, thereby neutralizing the activity of these drugs and reducing the effectiveness of treatment[26]. Second, Immune escape occurs: Tumor cells are sometimes able to escape attack by the immune system through a variety of mechanisms. In some cases, high levels of immunoglobulins may be a sign of overactivation of the immune system, and tumor cells may take steps to escape from the immune system[27]. Third, immune related adverse events take place: immunotherapy may cause immune related adverse events, including increased production of immunoglobulins. These adverse events may be related to treatment tolerance and prognosis[28]. More efforts will be required to elucidate the mechanism underlying ICIs-mediated survivals of NSCLC patients. Accumulating studies have revealed that high ANC will promote tumor progression and deterioration[29-31]A retrospective studyon the analysis of 88 patients with NSCLC treated with PD-1 inhibitors indicated that the lower the patient’s ANC at baseline, the better the response to treatment (P=0.017) [32]. In addition, it was found that high ANC before treatment was associated with poor prognosis in lung cancer patients treated with Anlotinib[33]. A descriptive review of immunotherapy for advanced renal cancer also stated that low ANC and NLR were associated with good prognosis of patients[34] . A predictive model for ICIs treatment of NSCLC patients has been established, including the impact of ANC and LDH and some clinical characteristics, ECOG PS score, BMI, etc. on prognosis[23] . Several reports indicated that the prognosis of tumor immunotherapy was related to NLR[35-37] , Although both ANC and NLR are related to immune status and the degree of inflammation, their biological significance may vary. In some cases, ANC may directly indicate immunosuppressive or inflammatory status, while NLR may suggest the balance between neutrophils and lymphocytes, and the prognostic impact of immunotherapy may be relatively complex. In the present study, we found that GLB, ANC, bone metastasis and PD-L1 were closely associated with the survival of NSCLC patients, and could be used as potential biomarkers for diagnosis of NSCLC patients with ICIs treatment. We look forward to following up this study to further investigate the different treatment markers for NSCLC patients, providing a basis for individualized treatment.
In the present study, we took into account the impact of distant organ metastasis on prognosis, and found that only bone metastasis was of statistical significance and predictive value in PFS and OS. We proposed that there might be several reasons. First, there were more patients with bone metastasis and fewer patients with other metastases such as liver, adrenal gland and brain, so the analysis results may be biased. Second, bone metastasis may affect the immune status of patients and the response to immunotherapy, which may lead to immunosuppression, inflammatory response and bone marrow fibrosis, thus affecting the effectiveness of immunotherapy. In addition, bone metastasis may also affect the prognosis of immunotherapy through other mechanisms, such as the change of tumor microenvironment[38]. Third, bone metastasis may affect the prognosis of lung cancer immunotherapy by affecting the bone marrow microenvironment and immune response. Thus, our result supported the previous study that bone metastasis may serve as a reliable indicator of prognosis for the patients with advanced NSCLC treated with ICIs[39].The prognostic factors of immunotherapy obtained by the model established in this study are somewhat different from those of previous publication, because we found that the expression of PD-L1 in tissues has statistical significance in both univariate and multivariate analysis in OS. Although it is still widely recognized standard for evaluating the efficacy of immunotherapy in patients, our data show that PD-L1 in PFS was only significant in univariate analysis, but not in multivariate analysis, indicating that the correlation between PD-L1 and PFS was not as strong as that of OS.
GLB was rarely used in the previous tumor prediction model. However, in this study,we found it was a potential biomarker of statistical significance to the survival of NSCLC patients. Importantly, our model in this study can perform better prediction by the score grouping of advantages and disadvantages factors or nomogram model.Ofnote, there are some limitations to this study, including the number of patients, most of the 123 patients treated with combination drugs, not ICIs monotherapy. Some of these drugs are not used for first-line treatment, and relevant information needs to be supplemented in the follow-up study to continue to analyze the predictive value for the same first-line treatment. Furthermore, the mechanism underlying the prognostic role of nomogram requires further efforts. In conclusion, our data show that the examination of ANC, GLB and bone metastasis can predict the PFS and OS of advanced/metastatic NSCLC patients treated with ICIs, and the optimal cut-off value can provide valuable reference for clinical practice. Conflict of interest, The authors declare that they have no conflict of interest.
This study was supported by Beijing Municipal Public Welfare Development and Reform Pilot Project for Medical Research Institutes(PWD&RPP-MRI),(No.JYY2024-14). Beijing Municipal Public Welfare Development and Reform Pilot Project for Medical Research Institutes (PWD&RPP-MRI),(No.JYY2023-15).
The current study was conducted with the approval of the Beijing Chest Hospital Medical Ethics Committee (YJS-2023-09)
1. Rebecca L Siegel , Angela N Giaquinto , Ahmedin Jemal Siegel R L, A.N. Giaquinto and A. Jemal, Cancer statistics, 2024. CA Cancer J Clin, 2024; 74(1): p. 12-49.
2. R S Zheng , R Chen , B F Han , S M Wang , L Li , K X Sun , H M Zeng Han B, et al., Cancer incidence and mortality in China, 2022. Journal of the National Cancer Center, 2024;
3. Parvarish Sharma , Meenu Mehta , Daljeet Singh Dhanjal , Simran Kaur , Gaurav Gupta ,Sharma P, et al., Emerging trends in the novel drug delivery approaches for the treatment of lung cancer. Chem Biol Interact, 2019; 309: p. 108720.
4. Martin Reck , Delvys Rodríguez-Abreu , Andrew G Robinson , Rina Hui , Tibor Csőszi Mok T, et al, Pembrolizumab versus chemotherapy for previously untreated, PD-L1-expressing, locally advanced or metastatic non-small-cell lung cancer (KEYNOTE-042): a randomised, open-label, controlled, phase 3 trial. Lancet, 2019; 393(10183): p. 1819-1830.
5. Scott J Antonia , Augusto Villegas , Davey Daniel , David Vicente , Shuji Murakami , Antonia SJ, et al., Durvalumab after Chemoradiotherapy in Stage III Non-Small-Cell Lung Cancer. N Engl J Med, 2017; 377(20): p. 1919-1929.
6. David R Spigel , Corinne Faivre-Finn , Jhanelle E Gray , David Vicente , David Planchard , Spigel DR, et al., Five-Year Survival Outcomes From the PACIFIC Trial: Durvalumab After Chemoradiotherapy in Stage III Non-Small-Cell Lung Cancer. J Clin Oncol, 2022; 40(12): p. 1301-1311.
7. Lingzhi Hong , Marcelo V Negrao, Seyedeh S Dibaj , Runzhe Chen , Alexandre Reuben Hong L, et al., Programmed Death-Ligand 1 Heterogeneity and Its Impact on Benefit From Immune Checkpoint Inhibitors in NSCLC. J Thorac Oncol, 2020; 15(9): p. 1449-1459.
8. Timothy S Fitzsimmons , Niharika Singh , Thomas D J Walker , Claire Newto Fitzsimmons, T.S, et al., Immune checkpoint inhibitors efficacy across solid cancers and the utility of PD-L1 as a biomarker of response: a systematic review and meta-analysis. Front Med (Lausanne), 2023; 10: p. 1192762.
9. D Ross Camidge , Robert C Doebele , Keith M Kerr Camidge DR, R.C. Doebele and K.M. Kerr, Comparing and contrasting predictive biomarkers for immunotherapy and targeted therapy of NSCLC. Nat Rev Clin Oncol, 2019; 16(6): p. 341-355.
10. Karthik Suresh , Jarushka Naidoo , Cheng Ting Lin , Sonye Danoff Suresh K, et al., Immune Checkpoint Immunotherapy for Non-Small Cell Lung Cancer: Benefits and Pulmonary Toxicities. Chest, 2018; 154(6): p. 1416-1423.
11. Ye Wang , Zhuang Tong , Wenhua Zhang , Weizhen Zhang , Anton Buzdin Wang Y, et al., FDA-Approved and Emerging Next Generation Predictive Biomarkers for Immune Checkpoint Inhibitors in Cancer Patients. Front Oncol, 2021; 11: p. 683419.
12. Deborah Blythe Doroshow , Sheena Bhalla , Mary Beth Beasley , Lynette M Sholl , Keith M Kerr Doroshow DB, et al., PD-L1 as a biomarker of response to immune-checkpoint inhibitors. Nat Rev Clin Oncol, 2021; 18(6): p. 345-362.
13. Biagio Ricciuti , Greg Jones , Mariano Severgnini , Joao V Alessi , Gonzalo Recondo Ricciuti B, et al., Early plasma circulating tumor DNA (ctDNA) changes predict response to first-line pembrolizumabbased therapy in non-small cell lung cancer (NSCLC). J Immunother Cancer, 2021; 9(3).
14. Nicolas Guibert , Myriam Delaunay , Amélie Lusque , Nadia Boubekeur , Isabelle Rouquette Guibert N, et al., PD-L1 expression in circulating tumor cells of advanced non-small cell lung cancer patients treated with nivolumab. Lung Cancer, 2018; 120: p. 108- 112.
15. Jing Huang , Hongjiang Pu , Jintao He , Xiaohu Tang Huang J, et al., Prognostic Value of the Lung Immune Prognostic Index for Metastatic Non-Small Cell Lung Cancer Patients: A Chinese Cohort Study. Int J Gen Med, 2023; 16: p. 881-893.
16. Wenxian Wang , Zhangzhou Huang , Zongyang Yu , Wu Zhuang , Weijun Zheng Wang W, et al., Prognostic Value of the Lung Immune Prognostic Index May Differ in Patients Treated With Immune Checkpoint Inhibitor Monotherapy or Combined With Chemotherapy for Non-small Cell Lung Cancer. Front Oncol, 2020; 10: p. 572853.
17. Wenxian Wang , Zhangzhou Huang , Zongyang Yu , Wu Zhuang , Weijun Zheng Prelaj A, et al., EPSILoN: A Prognostic Score Using Clinical and Blood Biomarkers in Advanced Non-Small-cell Lung Cancer Treated With Immunotherapy. Clin Lung Cancer, 2020; 21(4): p. 365-377.e5.
18. Miriam Möller , Steffi Turzer , Wolfgang Schütte , Barbara Seliger , Dagmar Riemann Moller M, et al., Blood Immune Cell Biomarkers in Patient With Lung Cancer Undergoing Treatment With Checkpoint Blockade. J Immunother, 2020; 43(2): p. 57-66.
19. Ou Yamaguchi , Kyoichi Kaira , Hisao Imai , Atsuto Mouri , Ayako Shiono , Yu Miura Yamaguchi O, et al., Clinical utility of inflammatory and nutritious index as therapeutic prediction of nivolumab plus ipilimumab in advanced NSCLC. Oncology, 2023.
20. Aya Nakaya , Takayasu Kurata , Hiroshige Yoshioka , Yuki Takeyasu, Maiko Niki Nakaya A, et al., Neutrophil-to-lymphocyte ratio as an early marker of outcomes in patients with advanced non-small-cell lung cancer treated with nivolumab. Int J Clin Oncol, 2018; 23(4): p. 634-640.
21. Man Zhang , Xiaolu Long , Yi Xiao , Jin Jin , Caixia Chen , Jiao Meng , Wanying Liu Zhang M, et al., Assessment and predictive ability of the absolute neutrophil count in peripheral blood for in vivo CAR T cells expansion and CRS. J Immunother Cancer, 2023; 11(11).
22. Si-Cong Ma , Xue Bai , Xue-Jun Guo Ma S.C, et al., Organ-specific metastatic landscape dissects PD-(L)1 blockade efficacy in advanced non-small cell lung cancer: applicability from clinical trials to realworld practice. BMC Med, 2022; 20(1): p. 120.
23. Si-Cong Ma , Xue Bai , Xue-Jun Guo Wang M, et al., Exploration of a novel prognostic model based on nomogram in non-small cell lung cancer patients with distant organ metastasis: implications for immunotherapy. Transl Lung Cancer Res, 2023; 12(10): p. 2040- 2054.
24. Jie Chen , Ye Zhou , Yu Xu , Hui-Yan Zh Chen J, et al., Low pretreatment serum globulin may predict favorable prognosis for gastric cancer patients. Tumour Biol, 2016; 37(3): p. 3905-11.
25. Wanyong Jin , Mo Zhu , Yang Zheng , Yuanyuan Wu , Xu Ding Jin W, et al., Perineural invasion, lactate dehydrogenase, globulin, and serum sodium predicting occult metastasis in oral cancer. Oral Dis, 2022; 28(1): p. 132-141.
26. Hany E Marei , Anwarul Hasan , Giacomo Pozzoli , Carlo Cenciarelli Marei H.E, et al., Cancer immunotherapy with immune checkpoint inhibitors (ICIs): potential, mechanisms of resistance, and strategies for reinvigorating T cell responsiveness when resistance is acquired. Cancer Cell Int, 2023; 23(1): p. 64.
27. Yu Rebecca Miao , Kaushik N Thakkar , Jin Qian , Mihalis S Kariolis , Wei Huang Miao Y.R, et al., Neutralization of PD-L2 is Essential for Overcoming Immune Checkpoint Blockade Resistance in Ovarian Cancer. Clin Cancer Res, 2021; 27(15): p. 4435-4448.
28. Sørensen A.S, et al., Tumor necrosis factor alpha neutralization attenuates immune checkpoint inhibitor-induced activation of intermediate monocytes in synovial fluid mononuclear cells from patients with inflammatory arthritis. Arthritis Res Ther, 2022; 24(1): p.
29. Zhu X, Y. Chen and Y. Cui, Absolute Neutrophil Count and Mean Platelet Volume in the Blood as Biomarkers to Detect Lung Cancer. Dis Markers, 2020; 2020: p. 1371964.
30. Akira Watanabe , Norihumi Harimoto , Kenichiro Araki , Norio Kubo , Takamichi Igarashi Watanabe A, et al., Absolute Neutrophil Count Predicts Postoperative Prognosis in Mass-forming Intrahepatic Cholangiocarcinoma. Anticancer Res, 2019. 39(2): p. 941-947.
31. Nomelini R, S. Rosekeila Simões Nomelini , Sâmela Daiere Soares Mota , Eddie Fernando Candido Murta Murta, Absolute band neutrophils count is a predictor of overall survival in advanced uterine cervical cancer. Arch Gynecol Obstet, 2022; 306(5): p. 1697- 1701.
32. Alona Zer , Mike R Sung , Preet Walia , Leila Khoja , Manjula Maganti Zer A, et al., Correlation of Neutrophil to Lymphocyte Ratio and Absolute Neutrophil Count With Outcomes With PD-1 Axis Inhibitors in Patients With Advanced Non-Small-Cell Lung Cancer. Clin Lung Cancer, 2018; 19(5): p. 426-434.e1.
33. Rong Chen , Fang-Ying Lu , Bing Liu , Jingwen Huang Chen R, et al., Absolute Neutrophil Count in the Peripheral Blood Predicts Prognosis in Lung Cancer Patients Treated with Anlotinib. Cancer Manag Res, 2021; 13: p. 3619-3627.
34. Matteo Rosellini , Andrea Marchetti , Veronica Mollica Rosellini M, et al., Prognostic and predictive biomarkers for immunotherapy in advanced renal cell carcinoma. Nat Rev Urol, 2023; 20(3): p. 133- 157.
35. D Viñal , L Gutierrez-Sainz , D Martinez , J A Garcia-Cuesta Vinal D, et al., Prognostic value of neutrophil-to-lymphocyte ratio in advanced cancer patients receiving immunotherapy. Clin Transl Oncol, 2021; 23(6): p. 1185-1192.
36. Adi Kartolo , Ryan Holstead , Sidra Khalid , Jeffrey Emack Kartolo A, et al., Serum neutrophil-to-lymphocyte ratio and platelet-tolymphocyte ratio in prognosticating immunotherapy efficacy. Immunotherapy, 2020; 12(11): p. 785-798.
37. Hui Ouyang , Bijing Xiao , Yan Huang , Zhiqiang Wang Ouyang H, et al., Baseline and early changes in the neutrophil-lymphocyte ratio (NLR) predict survival outcomes in advanced colorectal cancer patients treated with immunotherapy. Int Immunopharmacol, 2023; 123: p. 110703.
38. Lorenza Landi , Federica D’Incà , Alain Gelibter , Rita Chiari , Francesco Grossi Landi L, et al., Bone metastases and immunotherapy in patients with advanced non-small-cell lung cancer. J Immunother Cancer, 2019; 7(1): p. 316.
39. Alessandro Del Conte , Elisa De Carlo , Elisa Bertoli , Brigida Stanzione Del C.A, et al., Bone Metastasis and Immune Checkpoint Inhibitors in Non-Small Cell Lung Cancer (NSCLC): Microenvironment and Possible Clinical Implications. Int J Mol Sci, 2022; 23(12).
Yan Zhao, Tongmei Zhang and Guirong Wang. The GLB, ANC, Bone Metastasis And PD-L1 Are Potential Predictive Markers For The Prognosis Of Advanced Or Metastatic NSCLC Treated With Immune Checkpoint Inhibitors. Insights of Clinical and Medical Images 2024.